Example 4: Classification
Regression formulation
Let’s first treat the problem as a regression problem (output dimension = 1, MSE loss).
create the two moon dataset
from kan import *
import matplotlib.pyplot as plt
from sklearn.datasets import make_moons
import numpy as np
device = torch.device('cuda' if torch.cuda.is_available() else 'cpu')
print(device)
dataset = {}
train_input, train_label = make_moons(n_samples=1000, shuffle=True, noise=0.1, random_state=None)
test_input, test_label = make_moons(n_samples=1000, shuffle=True, noise=0.1, random_state=None)
dtype = torch.get_default_dtype()
dataset['train_input'] = torch.from_numpy(train_input).type(dtype).to(device)
dataset['test_input'] = torch.from_numpy(test_input).type(dtype).to(device)
dataset['train_label'] = torch.from_numpy(train_label[:,None]).type(dtype).to(device)
dataset['test_label'] = torch.from_numpy(test_label[:,None]).type(dtype).to(device)
X = dataset['train_input']
y = dataset['train_label']
plt.scatter(X[:,0].cpu().detach().numpy(), X[:,1].cpu().detach().numpy(), c=y[:,0].cpu().detach().numpy())
cuda
<matplotlib.collections.PathCollection at 0x7f3839ef1c10>
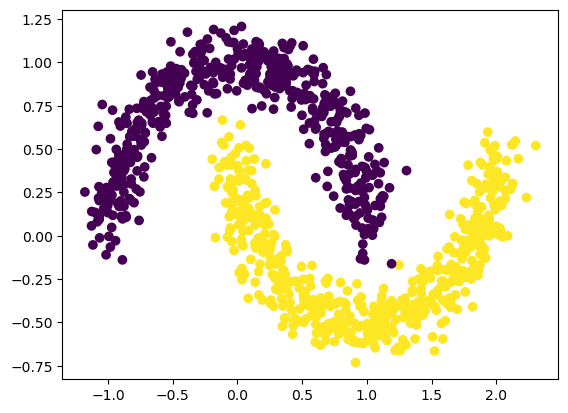
Train KAN
model = KAN(width=[2,1], grid=3, k=3, device=device)
def train_acc():
return torch.mean((torch.round(model(dataset['train_input'])[:,0]) == dataset['train_label'][:,0]).type(dtype))
def test_acc():
return torch.mean((torch.round(model(dataset['test_input'])[:,0]) == dataset['test_label'][:,0]).type(dtype))
results = model.fit(dataset, opt="LBFGS", steps=20, metrics=(train_acc, test_acc));
results['train_acc'][-1], results['test_acc'][-1]
checkpoint directory created: ./model
saving model version 0.0
| train_loss: 1.55e-01 | test_loss: 1.56e-01 | reg: 3.94e+00 | : 100%|█| 20/20 [00:01<00:00, 15.55it
saving model version 0.1
(1.0, 0.9980000257492065)
Automatic symbolic regression
lib = ['x','x^2','x^3','x^4','exp','log','sqrt','tanh','sin','tan','abs']
model.auto_symbolic(lib=lib)
formula = model.symbolic_formula()[0][0]
ex_round(formula, 4)
fixing (0,0,0) with sin, r2=0.9654733538627625, c=2
fixing (0,1,0) with x, r2=0.975755512714386, c=1
saving model version 0.2
\[\displaystyle - 0.853 x_{2} - 0.3885 \sin{\left(3.1242 x_{1} - 1.5464 \right)} + 0.7063\]
How accurate is this formula?
# how accurate is this formula?
def acc(formula, X, y):
batch = X.shape[0]
correct = 0
for i in range(batch):
correct += np.round(np.array(formula.subs('x_1', X[i,0]).subs('x_2', X[i,1])).astype(np.float64)) == y[i,0]
return correct/batch
print('train acc of the formula:', acc(formula, dataset['train_input'], dataset['train_label']))
print('test acc of the formula:', acc(formula, dataset['test_input'], dataset['test_label']))
train acc of the formula: tensor(0.9980, device='cuda:0')
test acc of the formula: tensor(0.9970, device='cuda:0')
Classification formulation
Let’s then treat the problem as a classification problem (output dimension = 2, CrossEntropy loss).
Create the two moon datatset
from kan import KAN
import matplotlib.pyplot as plt
from sklearn.datasets import make_moons
import torch
import numpy as np
dataset = {}
train_input, train_label = make_moons(n_samples=1000, shuffle=True, noise=0.1, random_state=None)
test_input, test_label = make_moons(n_samples=1000, shuffle=True, noise=0.1, random_state=None)
dataset['train_input'] = torch.from_numpy(train_input).type(dtype).to(device)
dataset['test_input'] = torch.from_numpy(test_input).type(dtype).to(device)
dataset['train_label'] = torch.from_numpy(train_label).type(torch.long).to(device)
dataset['test_label'] = torch.from_numpy(test_label).type(torch.long).to(device)
X = dataset['train_input']
y = dataset['train_label']
plt.scatter(X[:,0].cpu().detach().numpy(), X[:,1].cpu().detach().numpy(), c=y[:].cpu().detach().numpy())
<matplotlib.collections.PathCollection at 0x7f383010d5b0>
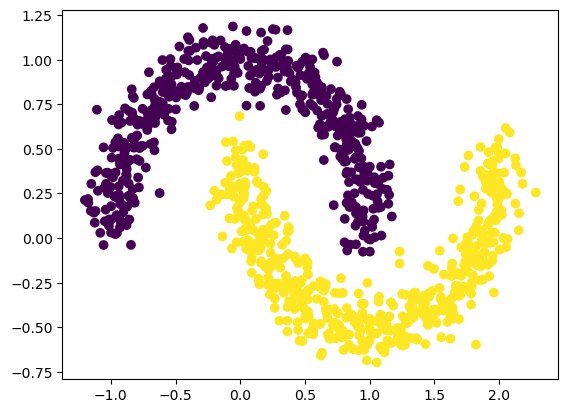
Train KAN
model = KAN(width=[2,2], grid=3, k=3, seed=2024, device=device)
def train_acc():
return torch.mean((torch.argmax(model(dataset['train_input']), dim=1) == dataset['train_label']).type(dtype))
def test_acc():
return torch.mean((torch.argmax(model(dataset['test_input']), dim=1) == dataset['test_label']).type(dtype))
results = model.fit(dataset, opt="LBFGS", steps=20, metrics=(train_acc, test_acc), loss_fn=torch.nn.CrossEntropyLoss());
checkpoint directory created: ./model
saving model version 0.0
| train_loss: 0.00e+00 | test_loss: 2.37e-01 | reg: 4.10e+02 | : 100%|█| 20/20 [00:01<00:00, 18.81it
saving model version 0.1
Automatic symbolic regression
lib = ['x','x^2','x^3','x^4','exp','log','sqrt','tanh','sin','abs']
model.auto_symbolic(lib=lib)
fixing (0,0,0) with x, r2=0.48220324516296387, c=1
fixing (0,0,1) with x, r2=0.3202315866947174, c=1
fixing (0,1,0) with x, r2=0.9358773231506348, c=1
fixing (0,1,1) with x, r2=0.9290410876274109, c=1
saving model version 0.2
formula1, formula2 = model.symbolic_formula()[0]
ex_round(formula1, 4)
\[\displaystyle - 15.0316 x_{1} + 177.9349 x_{2} - 63.0716\]
ex_round(formula2, 4)
\[\displaystyle 60.4718 x_{1} - 156.0295 x_{2} + 16.9\]
How accurate is this formula?
# how accurate is this formula?
def acc(formula1, formula2, X, y):
batch = X.shape[0]
correct = 0
for i in range(batch):
logit1 = np.array(formula1.subs('x_1', X[i,0]).subs('x_2', X[i,1])).astype(np.float64)
logit2 = np.array(formula2.subs('x_1', X[i,0]).subs('x_2', X[i,1])).astype(np.float64)
correct += (logit2 > logit1) == y[i]
return correct/batch
print('train acc of the formula:', acc(formula1, formula2, dataset['train_input'], dataset['train_label']))
print('test acc of the formula:', acc(formula1, formula2, dataset['test_input'], dataset['test_label']))
train acc of the formula: tensor(0.8870, device='cuda:0')
test acc of the formula: tensor(0.8810, device='cuda:0')