Ziming Liu
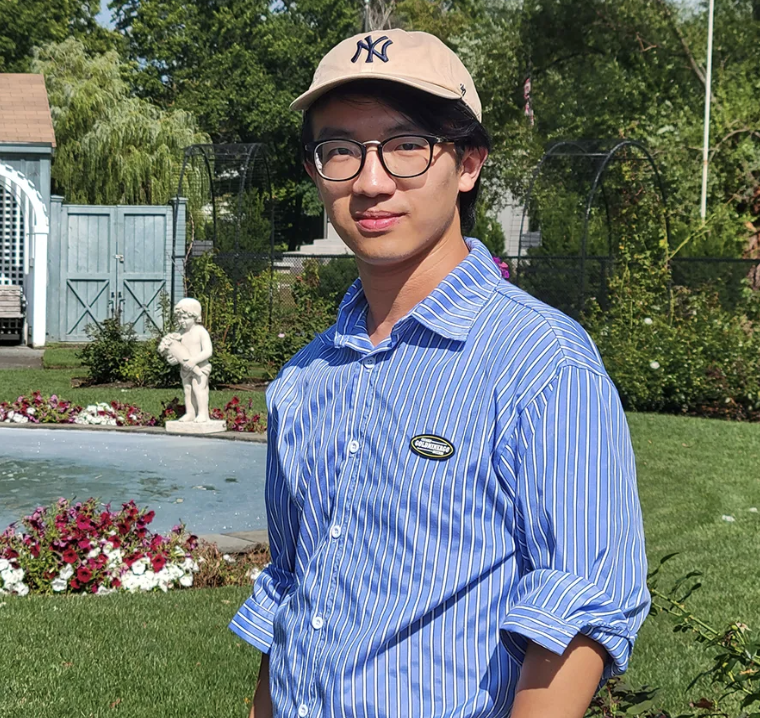
Ziming Liu (刘子鸣)
AI & Physics Researcher
PhD student @ MIT & IAIFI
Email: zmliu@mit.edu
I’m on the academic job market this year, looking for faculty and postdoc positions starting 2025 fall!
Hi! I’m Ziming, a final year PhD student at MIT & IAIFI, advised by Prof. Max Tegmark. Get my CV here. My research interests lie in the intersection of AI and physics (science in general):
- Science of AI: Understanding AI using science. I’m interested in understanding intriguing network phenomena (e.g., grokking, neural scaling laws) and their implications for next-generation AI.
- Science for AI: Advancing AI using science. I’m obsessed with building mathematical and scientific principles into AI.
- AI for Science: Advancing science using AI. I’m excited about inventing AI4Science models that are both accurate and interpretable.
I am grateful to the Quanta magazine (among other media outlets) which has covered all three branches of my research. Consider reading if you want to get some taste:
- Science of AI (Grokking): How Do Machines ‘Grok’ Data?
- Science for AI (Poisson Flow): The Physical Process That Powers a New Type of Generative AI
- AI for Science (KAN): Novel Architecture Makes Neural Networks More Understandable
My research philosophy is best described by John Hopfield’s inspiring words in his Nobel lecture:
[About physics] Physics is not defined by subject matter, but is a point of view that the world around us (with effort, ingenuity and adequete resources) is understandable in a predictive and reasonably quantitative fashion.
[About choosing problems] I am now looking for a big problem whose resolution and understanding will be of significance far beyond its normal disciplinary boundaries and will reorganize the fields from which they came.
To break disciplinary boundaries, I am decicated to bring young talents into this exciting field of AI + Science, as well as bring together AI researchers and domain scientists. I’m excited to teach courses on AI + Science when opportunities arise. Take a look at the syllabus for Science of AI and AI for Science that I’m designing and let me know if you would be interested in signup if such courses exist!
latest posts
May 27, 2024 | Philosophical thoughts on Kolmogorov-Arnold Networks |
---|---|
Jul 08, 2023 | Symbolic Regreesion? Structure Regression! |
Jun 16, 2023 | A Good ML Theory is Like Physics -- A Physicist's Analysis of Grokking |
selected publications
-
- Seeing is believing: Brain-inspired modular training for mechanistic interpretabilityEntropy, 2023
-
- The Clock and the Pizza: Two Stories in Mechanistic Explanation of Neural NetworksIn Thirty-seventh Conference on Neural Information Processing Systems , 2023
- Omnigrok: Grokking Beyond Algorithmic DataIn The Eleventh International Conference on Learning Representations , 2023
-
-
-